
We’ve been participating in the OCP Open Compute Project (OCP) Global Summit for many years, and while each year has brought pleasant surprises and announcements, as described in previous OCP blogs from 2022 and 2021, this year stands out in a league of its own. 2023 marks a significant turning point, notably with the advent of AI, which many speakers have referred to as a tectonic shift in the industry and a once-in-a-generation inflection point in computing and in the broader market. This transformation has unfolded within just the past few months, sparking a remarkable level of interest at the OCP conference. In fact, this year, the conference was completely sold out, demonstrating the widespread eagerness to grasp the opportunities and confront the challenges that this transformative shift presents to the market. Furthermore, at OCP 2023, there was a new track just to focus on AI. This year marks the beginning of a new era in the age of AI. AI is here! The race is on!
This new era of AI is marked and defined by the emergence of new generative AI applications and large language models. Some of these applications deal with billions and even trillions of parameters and the number of parameters seems to be growing 1000X every 2 to 3 years.
This complexity and size of the emerging AI applications dictate the number of accelerated nodes needed to run the AI applications as well as the scale and type of infrastructure needed to support and connect those accelerated nodes. Regrettably, as illustrated in the chart below presented by Meta at the OCP conference, a growing disparity exists between the requirements for model training and the available infrastructure to facilitate it.
This predicament poses the pivotal question: How can one scale to hundreds of thousands or even millions of accelerated nodes? The answer lies in the power of AI Networks purposively built and tuned for AI applications. So, what are the requirements that the AI Networks need to satisfy? To answer that question, let’s first look at the characteristics of AI workloads, which include but are not limited to the following:
- Traffic patterns consist of a large portion of elephant flows
- AI workloads require a large number of short remote memory access
- The fact that all nodes transmit at the same time saturates links very quickly
- The progression of all nodes can be held back by any delayed flow. In fact, Meta showed last year that 33% of elapsed time in AI/ML is spent waiting for the network.
Given these unique characteristics of AI workloads, AI Networks have to meet certain requirements such as high speed, low tail-latency, lossless and scalable fabrics.
In terms of high-speed performance, the chart below, which I presented at OCP, shows that by 2027, we anticipate that nearly all ports in the AI back-end network will operate at a minimum speed of 800 Gbps, with 1600 Gbps comprising half of the ports. In contrast, our forecast for the port speed mix in the front-end network reveals that only about a third of the ports will be at 800 Gbps speed by 2027, while 1600 Gbps ports will constitute just 10%. This discrepancy in port speed mix underscores the substantial disparity in requirements between the front-end network, primarily used to connect general-purpose servers, and the back-end network, which primarily supports AI workloads.
In the pursuit of achieving tail-latency and creating a lossless fabric, we are witnessing numerous initiatives aimed at enhancing Ethernet and modernizing it for optimal performance in AI workloads. For instance, the Ultra Ethernet Consortium (UEC) was established in July 2023, with the objective of delivering an open, interoperable, high-performance full-communications stack architecture based on Ethernet. Additionally, OCP has formed a new alliance to address significant networking challenges within AI cluster infrastructure. Another groundbreaking announcement from the OCP conference came from Google, who unveiled their opening of Falcon chips; a low-latency hardware transport, to the ecosystem through the Open Compute Project.
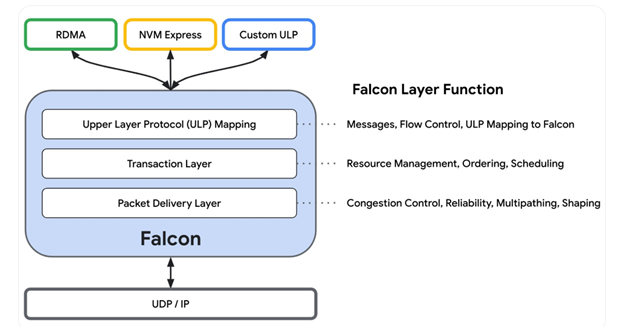
At OCP, there was a huge emphasis on adopting an open approach to address the scalability challenges of AI workloads, aligning seamlessly with the OCP 2023 theme: ‘Scaling Innovation Through Collaboration.’ Both Meta and Microsoft have consistently advocated, over the years, for community collaboration to tackle scalability issues. However, we were pleasantly surprised by the following statement from Google at OCP 2023: “A new era of AI systems design necessitates a dynamic open industry ecosystem”.
The challenges presented by AI workloads to network and infrastructure are compounded by the broad spectrum of workloads. As illustrated in the chart below showcased by Meta at OCP 2023, the diversity of workloads is evident in their varying requirements.
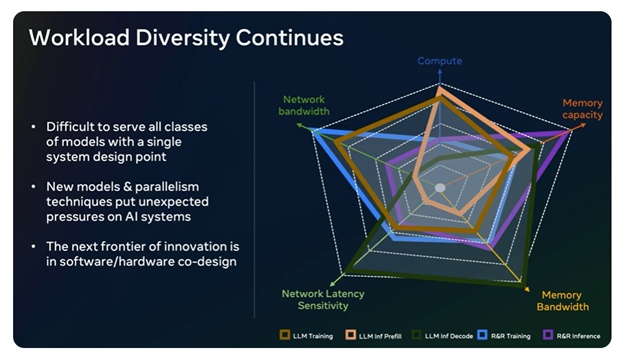
This diversity underscores the necessity of adopting a heterogeneous approach to build high-performance AI Networks and infrastructure capable of supporting a wide range of AI workloads. This heterogeneous approach will entail a combination of standardized as well as proprietary innovations and solutions. We anticipate that Cloud service providers will make distinct and unique choices, resulting in market bifurcation. In the upcoming Dell’Oro Group’s AI Networks for AI Workloads report, I delve into the various network fabric requirements based on cluster size, workload characteristics, and the distinctive choices made by cloud service providers.
Exciting years lie ahead of us! The AI journey is just 1% finished!